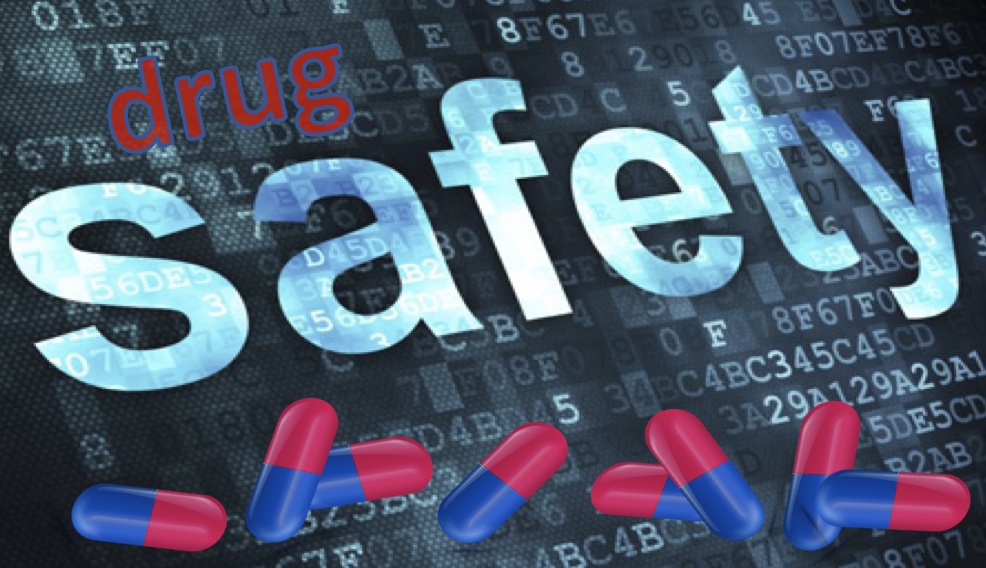
By Andreas Persidis, CEO Biovista Inc.
The increasing importance of predicting AEs & the role of Augmented Intelligence
Case in point: precision medicine and drug repurposing.
The approval of a drug has to date been the result of the careful assessment of its benefit-to-risk ratio. In this analysis, the benefit (efficacy) side of the equation has traditionally received much more attention (R&D funds) than the risk side (adverse events). But this benefit-to-risk “mix” is due for a change.
Firstly, there is an increasing social and market pressure for the pharma industry to predict potential side effects earlier and more accurately. Secondly, regulators are contemplating novel approval pathways where the part of the risk that is discharged during the pre/clinical trial stages is done so with higher confidence and specificity and with meaningful incentives for sponsors. Thirdly, pharma companies are slowly looking to leverage predicted adverse events (AEs) in a positive way i.e. not treat a predicted AE as a liability that reduces the possible market size of a new compound but as actionable insight that allows a more targeted development and hence the better differentiation of that compound.
But acting on predicted AEs is easier said than done. Efficacy is a positive outcome that is adequately assessed by the pre-clinical and clinical process; with powerful technologies and efficacious processes, it is relatively easy to get a clear answer to the question “does the new compound achieve the desired therapeutic effect or not?”. But the risk part has been much more difficult to ascertain, especially in cases where a more esoteric AE would require a larger population for it to be observed. In the case of AEs, we are trying to predict a negative outcome – meaning that an adverse event that fails to appear in the first year of a drug’s market availability is not guaranteed to not appear in the drug’s 2nd or 3rd year, as more patients get to use the new drug.
Moreover, once a mechanistic association is established between a specific drug’s target and some disease-related protein or pathway, whether the effect is beneficial or unwanted involves a nuanced value judgment that will determine whether one is looking at a drug repositioning opportunity, a predicted AE, or a sub-population at risk in a precision medicine context.
Such nuanced value judgments are typically not amenable to machine/deep learning approaches, since each case is different from the next and there are not many examples and large sets on which to train these algorithms. What promises to offer much better results is to adopt an augmented intelligence approach where technology assists human subject matter experts in three very important respects:
- being able to ask meaningful questions
- being able to identify, account for and assess the unique problem context
- creating hypotheses that can be readily justified in a peer review and expert group decision process